Australian Consumers Reap Benefits of Math Modeling Study
October 15, 1998
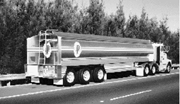
On its journey from refinery to customer, gasoline can be heated by the sun shining on a tank truck, cooled during storage in the station's underground tanks, heated again as it passes through pipes buried just below the sun-baked soil, and cooled again if it sits above ground in a shaded gas pump.
Paul Davis
Mel Gibson broke into the big time playing Mad Max, the hero of a movie about an Australian postnuclear society that treasured gasoline as if it were gold. Mad Max and his buddies never paused in their battles to ponder a fact of basic physics: The volume of gasoline, and hence its energy content, changes about 1% with every 9�C change in temperature. In Australia, a country so vast that it would stretch from Maine to Venezuela, a gallon of cold gas in the south might go a good deal farther than a warm one up north.
Concerns about the possibility of such inequities led in 1991 to a proposal that all retail gasoline sellers be required to install temperature compensation equipment. The basic question was whether the savings to consumers, who would eventually bear the costs of such changes, would outweigh the cost of buying and maintaining the compensation devices.
Laying a scientifically credible foundation for answering that question offered a starring role for the Mathematical and Information Sciences (CMIS) Division of CSIRO, the Commonwealth Scientific and Industrial Research Organisation. Rather than Mel Gibson's fast action and fantasy heroics, CSIRO staff members Graham Mills and Mark Westcott provided a monumental but meticulous four-year study for the Australian Institute of Petroleum (AIP). Decisions based on that work saved consumers and the oil industry enough to finance their own movie: The AIP-estimated expenditures would have been $A300 million for the purchase of temperature compensation devices and $A50 million per year for their operation.
The decision on correcting for temperature swung on the distribution all across Australia of gasoline temperatures at the point of retail sale. In a project supported by the AIP, Mills, Westcott, and their colleagues set out to determine the national averages of the sale and delivery temperatures to a preassigned degree of precision. They proceeded in two stages, first developing a methodology that promised reliable results at reasonable cost and then implementing the methodology.
The precision required for the temperature estimates was 2.7�C, corresponding to a volume error of 0.3%, the error in fuel dispensers allowed by government regulations. "It was the precision requirement that drove most of the subsequent development of methodology, for example determining how many samples we needed and how we should distribute them geographically," Westcott says. "It was also very much on the minds of the industry people on the project committee. If we recommended a methodology change for operational reasons, their first question was always, 'Will this affect the accuracy of the results?'"
Methodology
Gasoline begins its journey from refinery to Australian retail customer via ocean tanker (or road or rail or pipeline). Perhaps after a stop at an intermediate depot, it continues by truck to underground tanks at a service station, then passes through underground piping to the familiar gas pump, hose, and nozzle and into the customer's vehicle.
Along the way, the gasoline may be heated by the sun shining on a tank truck, cooled during storage in the station's underground tanks, heated again as it passes through pipes buried just below the sun-baked soil, and cooled again if it sits above ground in a shaded gas pump. The temperature of the fuel at the moment of retail sale depends on the liter-by-liter mix of gasoline at various temperatures from different points in the storage and delivery cycle.
The temperature of the gas is fairly easy to measure in the early stages of its journey, when volumes are large and transfers are relatively infrequent and through closed connections. Indeed, at one of these first steps, the volume of gasoline is temperature-corrected to 15�C in order to calculate excise tax. Gathering temperature data at the last step, the retail sale, is more problematic because transactions are frequent, involve smaller volumes, and use an open nozzle, for which temperature measurement is both difficult and dangerous.
These considerations led Mills and Westcott to a fundamental methodological decision: They would model and simulate sales at retail outlets but sample the deliveries to these outlets.
Implementing that choice led to formidable tasks of instrumentation, data collection, and modeling. Full instrumentation of a sample of retail stations without modeling, however, would have doubled the cost of the project, according to Noel Barton, science and industry manager for CMIS.
Sampling
To acquire data describing delivery temperatures and volumes at Australia's 9000 retail gas stations, a sample of 127 sites was selected to cover 16 ambient temperature strata and two strata of delivery volumes. Behind that design lay a host of practical issues: identifying stations, learning annual sale volumes (sensitive business information that is not readily disclosed), securing the participation of station operators, building a list of reserve sites to replace those that might become unavailable, and so on.
Over the data-collection period of 12 months, temperature and volume of two randomly chosen deliveries per season were recorded at each of the 127 sites. Engineering this data collection to ensure randomness was another logistic challenge, for the number of mobile test units was limited and Australia is a vast continent.
To support the modeling that related the temperature of the fuel at delivery to that at retail sale, three retail sites were selected for complete instrumentation. These stations were either new or in the process of being rebuilt, so that temperature, volume, and flow sensors could be installed throughout the underground storage and distribution system and in the sales pump, along with equipment for storing and transmitting these data. (The largest of these sites recorded 17 temperature and seven volume readings at different locations in the system at five-second intervals!)
Finally, five of the 127 delivery sample sites were instrumented to record sale volumes and temperatures as well. These five provided the test bed for validating the model relating delivery and sale temperatures.
Implementation
Actual implementation of this scheme required a level of organization that might seem more appropriate to fighting a war than to conducting a scientific experiment. Victory consisted of carrying out the work so that its results would be scientifically credible.
An outside contractor was responsible for sensor installation and data collection at the 127 sample sites (including travel all over the continent to measure delivery temperatures), as well as for the storage, transmission, and processing of the data. Mills and Westcott chose to hire a project manager, a kind of field general, to act as a liaison between the gas station operators and the data technicians. They also secured the cooperation of John Connolly, a colleague from CSIRO's Division of Telecommunications and Industrial Physics, to calibrate the many sensors needed in the field before and after the year of data collection.
Managing such a mass of data collected from all over Australia---ensuring the validity of the data received, processing the data according to agreed protocols, replacing faulty sensors, and so on---was itself a formidable task. For example, one site briefly lost data when its computer was accidentally unplugged during air-conditioning repairs. Longer gaps occurred elsewhere when rain flooded sensor wells and when a car destroyed a gas pump.
Mills and Westcott even had to derive station layouts from informal sketches prepared by the technicians measuring delivery temperatures at the 127 sample sites. They report that "most of the initial sketches needed some revision, though we did enjoy a number of extremely accurate and artistic entries!"
Modeling
Modeling problems arose on every scale. At the lower end, Mills and Westcott needed to account for a lag due to heat flow transients in the response of the temperature sensors used in the dispenser nozzles. The relation between the true fuel temperature and that measured by the sensor is governed by the simple linear first-order differential equation that expresses Newton's law of cooling. Once the cooling constant of the temperature probe has been obtained, the continuous relation between the two temperatures is completely known.
However, the probe temperature is measured at five-second intervals, not continuously. Using the cooling law differential equation to find the true temperature would require numerical differentiation, producing "notoriously noisy results," as Mills and Westcott described the familiar computational difficulty. Fortunately, what the investigators actually needed was the volume-averaged temperature, which can be derived smoothly and reliably by replacing the integration involved with sums over the five-second time steps.
Modeling temperature variation within an individual service station presented a larger-scale problem, incorporating the influences of deliveries, the underground tank and piping, the dispenser pump, the configuration of the individual station, and the pattern of sales. The last is important because each sale draws some quantity of gasoline through the system, there---by changing the mix of temperatures.
Aided by their colleague David Gates, Mills and Westcott discovered that they could accurately model a given heavily instrumented station like one of the three well-studied retail outlets, but they could not reliably transfer the model to another site for which less information was available. They were thus forced to resort "to statistical estimation of model relationships to a greater extent than we had planned."
Temperature behavior in the underground tanks at the stations presented another large-scale modeling challenge. A simple mixing formula accounted for the change in tank temperature after a delivery, but tank temperatures did not tend toward the asymptotic value determined solely by the ground temperature. Mills and Westcott suspected some subtle interaction with ground temperature near the tank but concluded that a detailed model of the corresponding heat transfer was not worthwhile.
Instead, they used regression to fit a sinusoidally varying asymptotic tank temperature. Its mean, phase, and amplitude were estimated from annual temperature data (collected from the nearest weather station, ideally at three-hour intervals) and from delivery data. This approach provided reliable tank temperature data for the test stations.
Studies of more complicated thermal or mixing relations at other points in the system produced no significant improvements in the results. Mills and Westcott also explored the sensitivity of predicted mean sale temperatures, the goal of their modeling and simulation work. They found the mean sale temperature value to be relatively insensitive to the thermal response times of both the underground tank and the gas pump. The most influential parameter appeared to be the mean tank temperature, which the investigators had modeled reliably by fitting the sinusoidal temperature profile.
Predicting Sale Temperatures
To characterize sale temperatures at a given station, a site-specific station model was coupled with ambient temperature data, a description of delivery temperatures and volumes based on the sampled deliveries, an underground tank temperature model, the dispenser and piping system response, and a realization of customer arrivals derived from a time-dependent Poisson process.
The target tolerance for mean sale temperatures was 2.7�C. For the eight well-instrumented stations, the combination of modeling and simulation predicted mean sale temperatures that differed from measured temperatures by at most 1.5�C, significantly better than the required precision!
To generate estimated sale temperatures for the 122 stations where only delivery data were measured, a site-specific version of the model was exercised for each station for the equivalent of one full year. The resulting simulated mean sale temperature, standard error, and temperature-volume profile were then used to predict average sale temperatures nationally with 95% confidence, as well as by state and territory.
The Bottom Lines
The result of this extensive modeling and simulation warmed the hearts of AIP members in several ways. "Our study gave reliable average values for AIP to use as the basis of their national average cost calculations," Westcott explains. "It showed also that there is little difference on average between delivery and sale temperatures."
Ideally, the study also would have provided a highly reliable picture of the variation about the mean of sale temperature. Westcott says, "AIP were very keen originally to get this profile with a high degree of precision, but reconsidered when we suggested what this might cost!" In the end, the industry was able to argue successfully from the results of the study that, on average, a national program of temperature correction was uneconomic.
AIP received this valuable information for a relatively modest investment, a fraction of the cost of fully instrumenting a sample of 127 retail stations and an even smaller proportion of the $A300 million estimated cost of temperature correction equipment. Ewen Macpherson, manager of government and public policy for AIP, reported pointedly that "the Ministerial Council on Consumer Affairs relied heavily on the CSIRO report and decided that it would not proceed with mandatory temperature compensation."
Noel Barton cites this project as an example of the ways in which "mathematics can be staggeringly cost effective," both through the 50% savings in project costs accounted for by the modeling component and through the national impact of the report as a whole, "an $A800 million benefit in total over ten years." The entire CSIRO study cost about $A2 million; Barton estimates that about $A400,000 of that amount was devoted to modeling and simulation.
Mills and Westcott may not have matched the fictitious adventures of Mad Max as he drove all over the Australian continent in search of gasoline, but they certainly overcame a wide range of real challenges-physical and statistical modeling, data collection and analysis, simulation, prediction, and validation-while settling the question of variation in sale temperatures. Their story had an ending that petroleum policy makers could believe, an ending that saved some very real Australian dollars.
Paul Davis is a professor in the Department of Mathematical Sciences at Worcester Polytechnic Institute.